Common Data Issues Faced by Businesses (SOLVED)
Effective data management remains a key challenge for many businesses. Fragmentation, limited access, and poor data quality hinder decision-making and process optimization. Below, we explore common data issues within companies and highlight the solutions developed by Cloud Levante’s data analysts, including ETL and Data Lakes, among others.
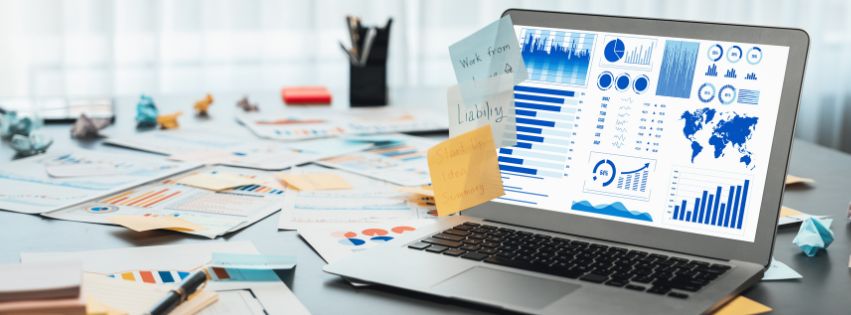
Fragmented Data
Issue: Customer information is scattered across various platforms (CRM, social media, website), preventing a unified view and making it difficult to provide a consistent customer experience.
Solution: Implement a Master Data Management (MDM) platform that centralizes data from different sources. Complement this with a data lake to store large volumes of unstructured data and process it to gain valuable insights, integrating ETL processes for synchronization and consolidation of information.
Outdated Data
Issue: The lack of updates to customer information leads to the use of obsolete data, affecting the effectiveness of communications and wasting resources on failed campaigns.
Solution: Implement data quality tools that perform automatic updates periodically, combined with continuous validation processes. If the data comes from multiple sources, a data lake helps capture historical data and maintain updated versions for easy comparison and updates.
Difficulty Accessing Data
Issue: Gaining access to critical data is complicated and requires the involvement of multiple teams, delaying strategic decision-making.
Solution: Deploy a self-service architecture for data, combining role-based access control (RBAC) systems with centralized governance.
Lack of Integration Between Systems
Issue: Inventory, sales, and reservation systems are not integrated, leading to data inconsistencies, inventory mismatches, and poor resource planning.
Solution: Implement middleware or integration solutions that connect different systems in real time. Additionally, a data lake can serve as a convergence point for all data from various systems, ensuring that any analysis process has consolidated and updated information.
Data Quality Issues
Issue: Incorrect or incomplete data in databases, such as erroneous addresses or contact information, result in delays and cost overruns in the supply chain.
Solution: Implement data validation and cleaning tools that automatically identify errors. Moreover, deduplication and enrichment processes can be applied. A data quality-focused approach will help improve the integrity and reliability of the information.
Lack of Data Governance
Issue: The absence of clear policies for data access exposes the company to security breaches and the exposure of sensitive information.
Solution: Define data governance policies that include permissions, access audits, and encryption of sensitive information. Utilize systems that enable robust access control to protect sensitive data, ensuring that only authorized personnel have access to relevant information.
Difficulty Analyzing Data
Issue: Data is disorganized, stored in various and dispersed formats, making efficient analysis difficult and delaying decision-making.
Solution: Standardize data formats through ETL processes. Additionally, consider a data lake for storing large volumes of data in their original formats. Using big data tools allows for processing that information at scale, extracting valuable insights more efficiently.
Inability to Scale
Issue: The data infrastructure does not support the rapid growth of the company, resulting in slow response times and making real-time analysis impossible.
Solution: Migrate to a cloud infrastructure with horizontal scalability. This allows for handling increased data volume seamlessly, ensuring that the company can continue to grow without interruptions in data analysis.
Data challenges in businesses are common but not insurmountable. With the right solutions, such as ETL solutions, self-service architectures, and the strategic use of data lakes, companies can address issues of fragmentation, access, quality, and scalability. This not only enhances operational efficiency but also improves strategic decision-making, optimizing data utilization at all levels.